Poplar is an important woody biomass crop and at the same time the model of choice for molecular research in trees. Although there is steady progress in resolving the functions of unknown genes, the identities of most secondary metabolites in poplar remain unknown. The lack of metabolite identities in experimental systems is a true gap in information content, and impedes obtaining deep insight into the complex biology of living systems. The main reason is that metabolites are difficult to purify because of their low abundance, hindering their structural characterization and the discovery of their biosynthetic pathways. In this ERC-funded project, we use CSPP, an innovative method recently developed in our lab, to systematically predict the structures of metabolites along with their biosynthetic pathways in poplar wood, bark and leaves. This CSPP method is based on a combination of metabolomics and informatics. In a next step, the CSPP tool is combined with two complementary genetic approaches based on genome sequences from 750 Populus nigra trees to identify the genes encoding the enzymes in the predicted pathways. GWAS identify SNPs in the genes involved in the metabolic conversions. Subsequently, rare defective alleles will be identified for these genes in the sequenced population. Genes identified by both approaches will then be further studied either by crossing natural poplars that are heterozygous for the defective alleles, or by CRISPR/Cas9-based gene editing in poplar. The functional studies are further underpinned by enzyme assays. Given that almost no information is currently available on the structure of most secondary metabolites, neither on their metabolic pathways, this large-scale identification effort will stimulate a quantum leap in our basic understanding of secondary metabolism in the tree model system poplar, and will lay the foundation to further develop poplar as an industrial wood-producing crop. We delivered proof-of-concept of this approach in Arabidopsis (Brouckaert et al., 2023).
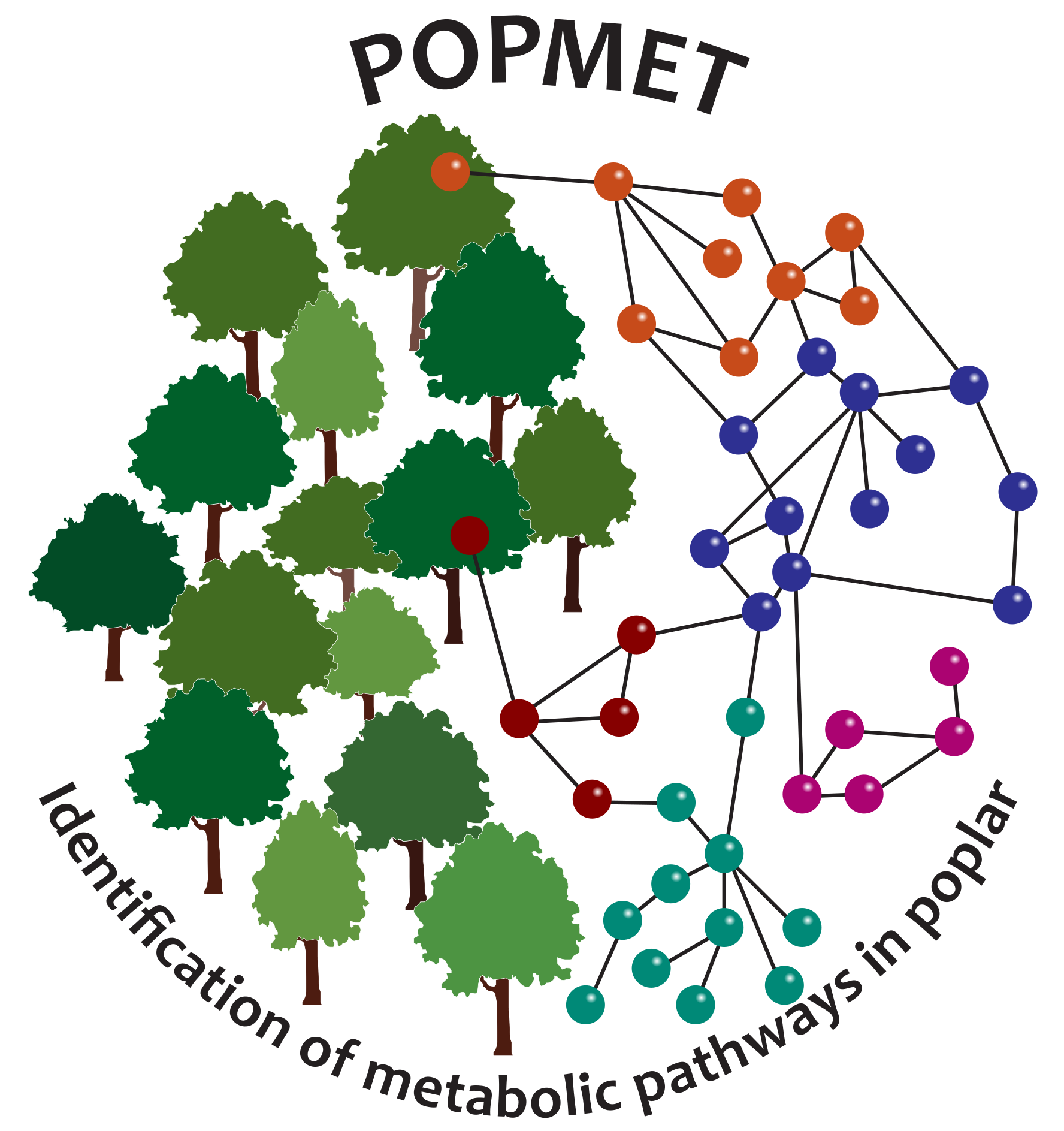