A main bottleneck in our gene discovery studies is that the identity of most metabolites is unknown. We can only understand the response to pathway perturbations, when we know the identity of the differentially accumulating compounds, e.g. in reverse genetics studies where we aim at identifying the substrate for an enzyme. To identify the structures of specialized metabolites in Arabidopsis, maize and poplar, the focal species in our reverse genetic analyses, we use the LCMS-based Candidate Substrate Product Pairs (CSPP) algorithm that was developed in our team and that is used to characterize unknown compounds (Morreel et al., 2014; Desmet et al., 2020; 2021). The algorithm searches for peak pairs that differ by a mass that corresponds to an enzymatic reaction. If this search is performed for all peaks in a chromatogram, and for the most prominent reactions that take place in metabolism, self-propagating networks are generated where each node is a metabolite and each edge a metabolic conversion. At the same time, the algorithm also predicts tentative biosynthetic pathways. In the ERC project ‘POPMET; Large-scale identification of secondary metabolites, their biosynthetic pathways and their genes in the model tree poplar’, we integrate mass spectrometry, systems biology, GWAS and reverse genetics to identify new genes in specialized metabolic pathways in poplar (see below).
Our expertise in metabolite profiling of secondary metabolites has allowed establishing the VIB Metabolomics Core facility (https://corefacilities.vib.be/metabcore).
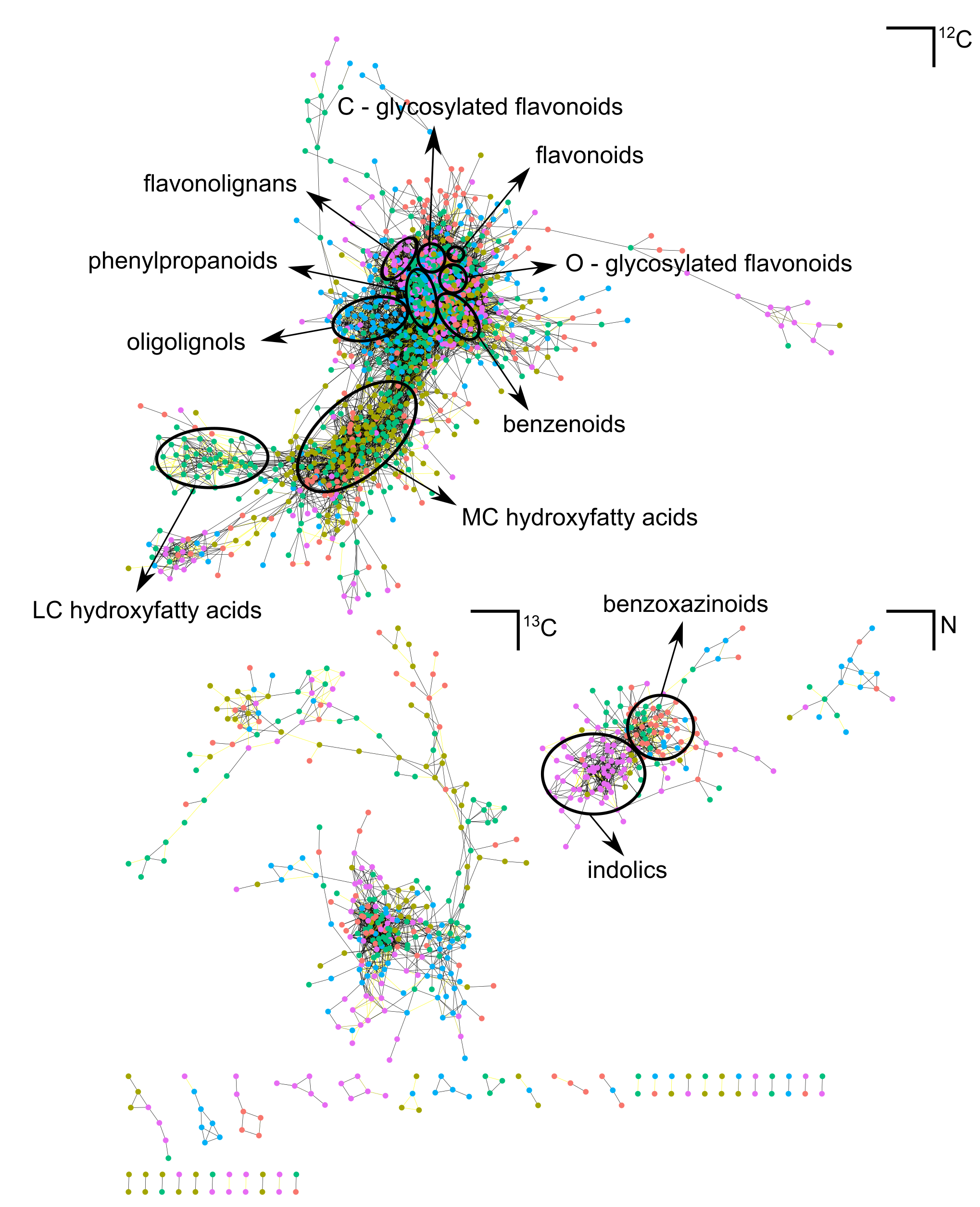